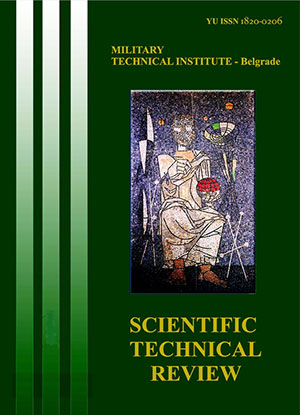
ISSN: 1820-0206
First published in
1950
About the journal
Editorial board
All
issues
Latest issue
Issue in progress
Publishing
Policy
Guide for authors
Reviwers guidelines
Submit your article
Contact
|
doi:10.5937/OTEH2402059N
Vol. 74, No.2 (2024), Pages:
59-64
Impressum
Contents
Simple Energy Detector for Two-Stage Classification for
Antidrone Systems
Snežana Zurovac
Nikola Petrović
Vasilija Joksimović
Ivan Pokrajac
Darko Mikanović
Boban Sazdić-Jotić
Signal detection theory, a fundamental concept in various scientific
disciplines, involves mandatory measuring of the signal features.
This theory finds applications in telecommunications, radar
technology, medical devices, automation and process control,
geophysical research, biometric systems, and security systems,
emphasizing its broad significance. Likewise, drone detection in the
radio-frequency domain is necessary for signal detection and ensures
efficient and reliable communication, surveillance, and security.
The recent conflict between Russia and Ukraine has underscored the
crucial role of drones in modern warfare. Our research can improve
the detection of any malicious drones that pose a threat, thereby
underlining the significance of the proposed methodology in modern
electronic warfare. This is a specialized approach to drone signal
detection based on two-stage classification with two key components:
a method based on spectrogram energy detection and deep learning
classification. Energy detection on the spectrograms is particularly
effective when the signal's energy characteristics differ
significantly from the surrounding noise. The practical
applicability of our proposed method was evaluated using the
publicly available VTI_DroneSET dataset, which contains a diverse
range of signals from three types of drones. Furthermore, we
conducted tests with the VTI_DroneUSRP dataset and signals from the
NI-USRP-2954 receiver, demonstrating the effectiveness and
practicality of the proposed method in real situations. The
successful detection and identification of Wi-Fi, Bluetooth, and
drone signals in both ISM frequency bands were performed, proving
the method's reliability. The proposed approach improved execution
times and energy savings, indicating that applying the energy
detector on the spectrograms in a two-stage classification
significantly enhances the performance of ADRO applications for
real-time drone detection. Furthermore, we conducted a comparative
analysis of different deep learning algorithms at the outset of
two-stage classification, which is a potential basis for adopting
this approach.
Key words: artificial intelligence, deep learning, detection,
drone, energy detector, receiver.
DOWNLOAD PDF
|